import tensorflow as tf mnist = tf.keras.datasets.mnist (x_train, y_train),(x_test, y_test) = mnist.load_data() x_train, x_test = x_train / 255.0, x_test / 255.0 model = tf.keras.models.Sequential([ tf.keras.layers.Flatten(input_shape=(28, 28)), tf.keras.layers.Dense(128, activation='relu'), tf.keras.layers.Dropout(0.2), tf.keras.layers.Dense(10, activation='softmax') ]) model.compile(optimizer='adam', loss='sparse_categorical_crossentropy', metrics=['accuracy']) model.fit(x_train, y_train, epochs=5) model.evaluate(x_test, y_test)
借助机器学习解决现实问题
查看相关示例,了解 TensorFlow 如何用于推进研究并构建 AI 赋能的应用。
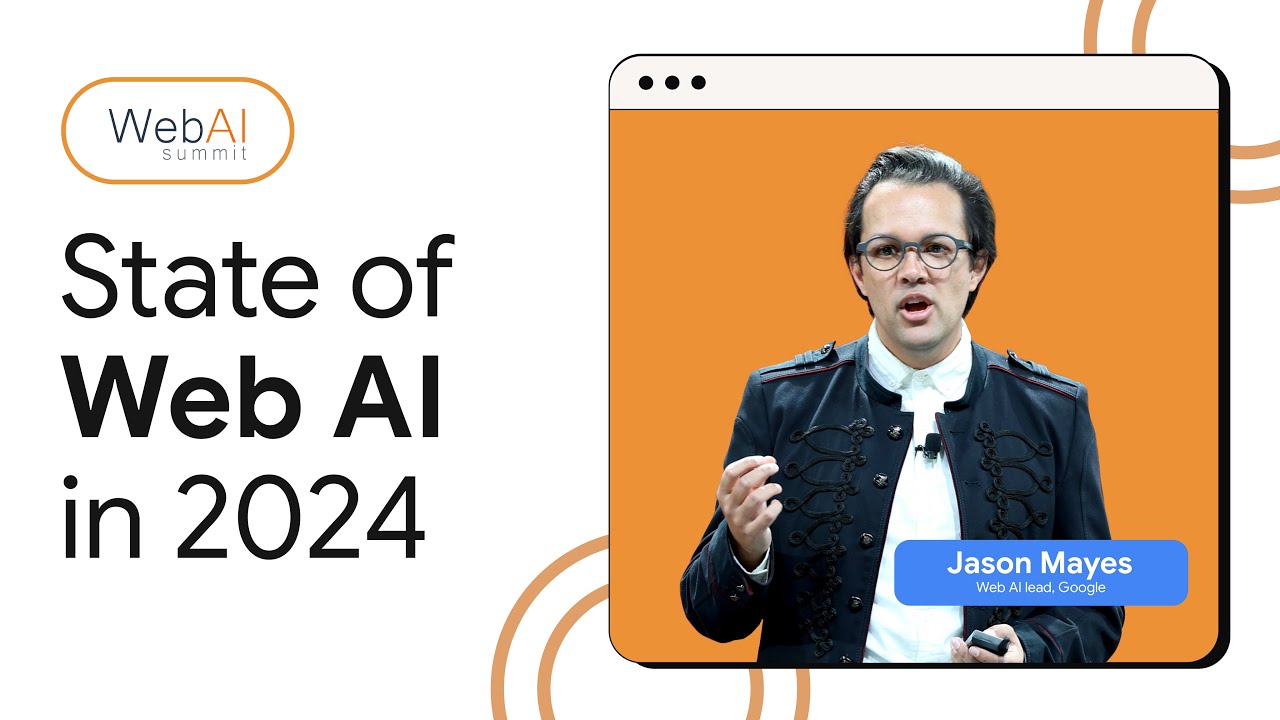
Explore the latest advancements in running models client-side with speakers from Chrome, MediaPipe, Intel, Hugging Face, Microsoft, LangChain, and more.

GNNs can process complex relationships between objects, making them a powerful technique for traffic forecasting, medical discovery, and more.
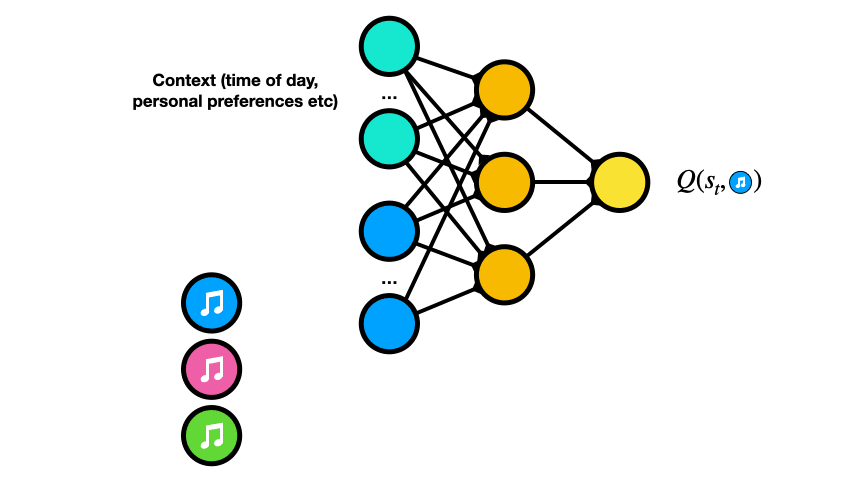

TensorFlow 有哪些新变化
阅读 TensorFlow 团队和社区发布的最新公告。
探索生态系统
探索经过生产环境测试的工具,加快建模、部署和其他工作流。
-
Library
TensorFlow.js
Train and run models directly in the browser using JavaScript or Node.js.
-
Library
LiteRT
Deploy ML on mobile and edge devices such as Android, iOS, Raspberry Pi, and Edge TPU.
-
API
tf.data
Preprocess data and create input pipelines for ML models.
-
Library
TFX
Create production ML pipelines and implement MLOps best practices.
-
API
tf.keras
Create ML models with TensorFlow's high-level API.
-
Resource
Kaggle Models
Find pre-trained models ready for fine-tuning and deployment.
-
Resource
TensorFlow Datasets
Browse the collection of standard datasets for initial training and validation.
-
Tool
TensorBoard
Visualize and track development of ML models.