推荐系统
从订餐到视频点播和音频在线播放,再到时尚内容, 推荐系统可为当今一些极受欢迎的应用提供助力。探索如何利用 TensorFlow 生态系统中的开源库和工具 构建可用于生产环境的推荐系统。
推荐系统通过提供最合乎用户需求的内容,提高用户 在您的应用内的互动度并提升用户体验。新型 Recommender 系统十分复杂,通常会细分为多个阶段, 以便在生产环境中实现低延迟。通过检索、 排序和可能的排序后阶段,系统会从大量候选项中 逐渐过滤掉不相关的推荐项,最终呈现用户最有 可能进行互动的一系列选项。
开始使用 TensorFlow Recommenders 进行构建, 这是一个简单易用的框架,可在 Recommender 系统的整个构建流程(从数据准备到部署)中发挥作用。
完成模型训练后,您可以将模型部署到生产环境中,以向最终用户提供推荐内容。TensorFlow Serving 可将模型投入生产环境,实现高性能推断。该平台旨在最大限度提高机器学习模型的吞吐量,并且能够为需要分布式服务的大型推荐模型提供支持。
# Deploy the retrieval model with TensorFlow Serving docker run -t --rm -p 8501:8501 \ -v "RETRIEVAL/MODEL/PATH:/models/retrieval" \ -e MODEL_NAME=retrieval tensorflow/serving & # Retrieve top movies that user 42 may like curl -X POST -H "Content-Type: application/json" \ -d '{"instances":["42"]}' \ http://localhost:8501/v1/models/retrieval:predict # Output # { # "predictions":[ # { # "output_1": [2.032, 1.969, 1.813], # "output_2": ["movie1”, “movie2”, “movie3”] # } # ] # } # Deploy the ranking model with TensorFlow Serving docker run -t --rm -p 8501:8501 \ -v "RANKING/MODEL/PATH:/models/ranking" \ -e MODEL_NAME=ranking tensorflow/serving & # Get the prediction score for user 42 and movie 3 curl -X POST -H "Content-Type: application/json" \ -d '{"instances":[{"user_id":"42", "movie_title":"movie3"}]}' \ http://localhost:8501/v1/models/ranking:predict # Output: # {"predictions": [[3.66357923]]}
改进推荐引擎的检索和排序阶段
大型推荐系统需要通过检索和排序阶段,以有效且高效的方式从数百万个候选项中确定最相关的推荐项。您可以使用 ScaNN 库中先进的近似最邻近 (ANN) 搜索算法和 TensorFlow Ranking 库中的排序学习 (LTR) 技术对 TensorFlow Recommenders 进行补充,提升推荐内容的契合度。
TensorFlow Ranking 是一个用于开发可扩容的神经 LTR 模型的库。该库提供了额外的功能来对候选推荐项进行排序,以便最大限度发挥排序实用程序的效用。
优化大型嵌入以进行模型训练和推断
嵌入查询操作是大型推荐系统的关键组成部分。利用硬件加速和动态嵌入技术,克服大型嵌入表中常见的性能瓶颈。
保护用户隐私
传统推荐引擎依赖于收集用户互动日志和基于原始用户活动来训练推荐模型。您可以整合 Responsible AI 开发实践,确保用户数据保持私密状态。
TensorFlow Federated 是一个可用于对分散式数据进行联邦学习和其他计算的框架。Federated Reconstruction 可将矩阵分解引入联邦学习设置,并且能够在推荐时更好地保护用户隐私。
为更复杂的 Recommender 采用先进的技术
虽然传统的协同过滤模型在业界得到广泛运用,但采用强化学习和图神经网络 (GNN) 等先进技术来构建推荐系统这一趋势日益明显。
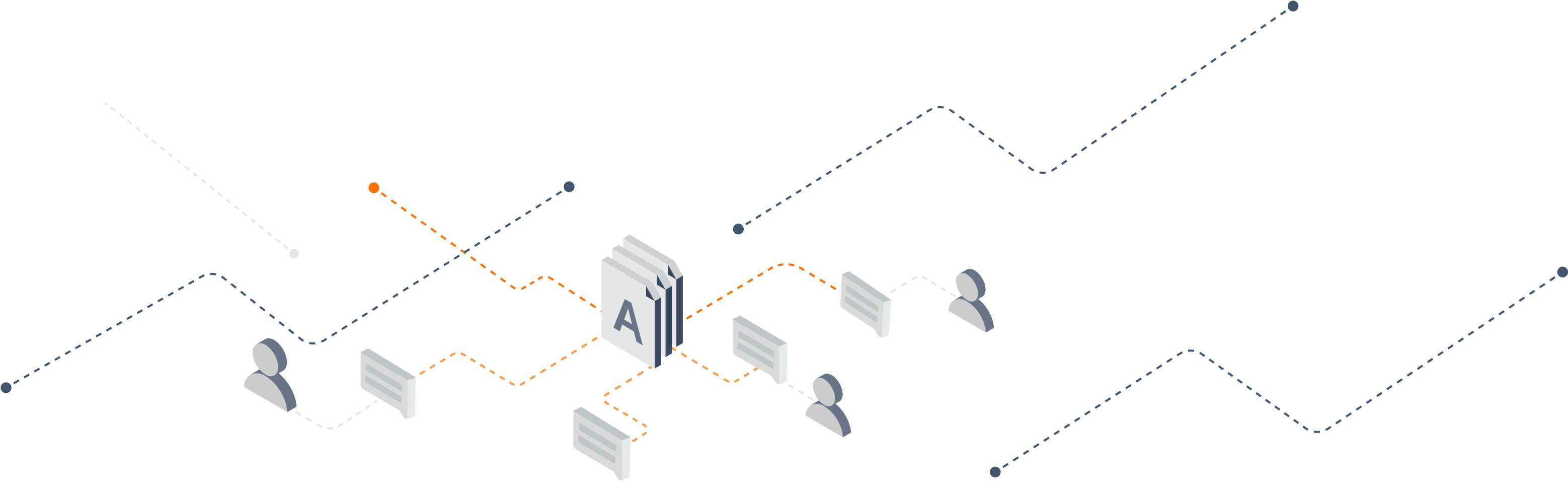
参考先进的推荐模型
如需对知名模型进行性能基准测试或自行构建推荐模型,请参阅热门模型(例如 NCF、DLRM 和 DCN v2)的官方 TensorFlow 实现,以遵循最佳实践。
教育资源
跟随分步讲解的课程和视频,进一步了解如何构建推荐系统。
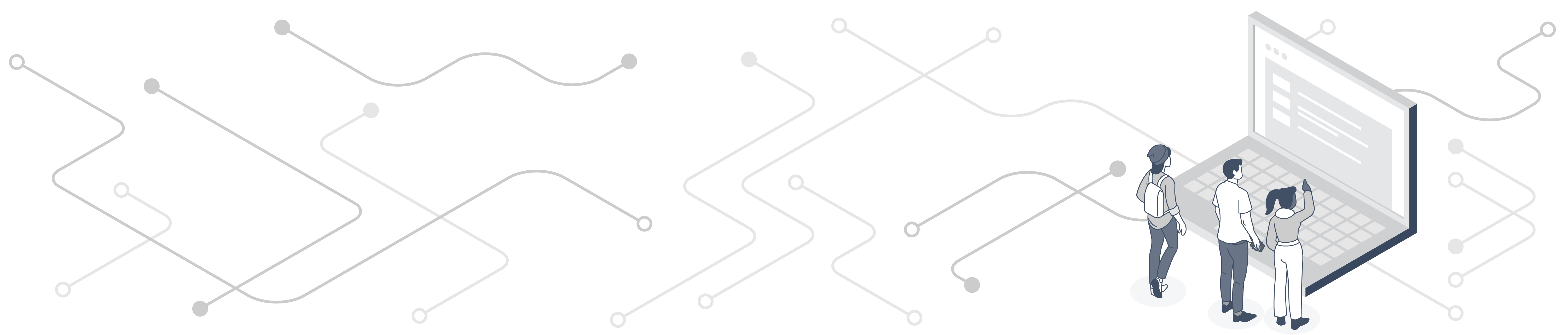
实际推荐系统
推荐系统可为各行各业中的应用提供助力,欢迎探索相关示例和案例研究。