# Install TF-DF !pip install tensorflow tensorflow_decision_forests # Load TF-DF import tensorflow_decision_forests as tfdf import pandas as pd # Load a dataset in a Pandas dataframe. train_df = pd.read_csv("project/train.csv") test_df = pd.read_csv("project/test.csv") # Convert the dataset into a TensorFlow dataset. train_ds = tfdf.keras.pd_dataframe_to_tf_dataset(train_df, label="my_label") test_ds = tfdf.keras.pd_dataframe_to_tf_dataset(test_df, label="my_label") # Train a Random Forest model. model = tfdf.keras.RandomForestModel() model.fit(train_ds) # Summary of the model structure. model.summary() # Compute model accuracy. model.compile(metrics=["accuracy"]) model.evaluate(test_ds, return_dict=True) # Export the model to a SavedModel. model.save("project/model")
# Install YDF !pip install ydf -U import ydf import pandas as pd # Load a dataset with Pandas ds_path = "https://raw.githubusercontent.com/google/yggdrasil-decision-forests/main/yggdrasil_decision_forests/test_data/dataset/" train_ds = pd.read_csv(ds_path + "adult_train.csv") test_ds = pd.read_csv(ds_path + "adult_test.csv") # Train a Gradient Boosted Trees model model = ydf.GradientBoostedTreesLearner(label="income").train(train_ds) # Look at a model (input features, training logs, structure, etc.) model.describe() # Evaluate a model (e.g. roc, accuracy, confusion matrix, confidence intervals) model.evaluate(test_ds) # Generate predictions model.predict(test_ds) # Analyse a model (e.g. partial dependence plot, variable importance) model.analyze(test_ds) # Benchmark the inference speed of a model model.benchmark(test_ds) # Save the model model.save("/tmp/my_model") # Export the model as a TensorFlow Saved Model model.to_tensorflow_saved_model("/tmp/my_saved_model")
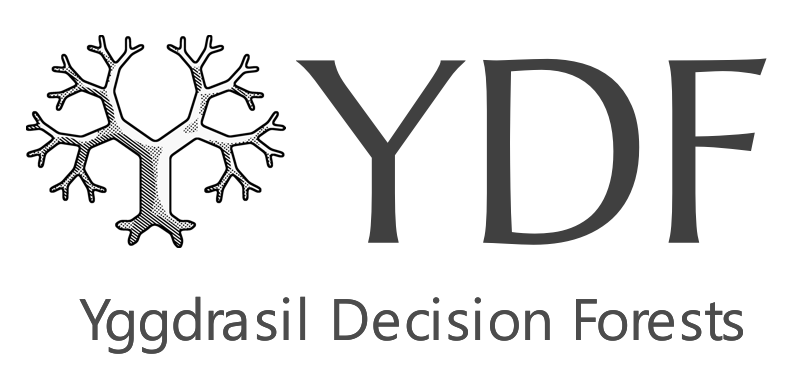
YDF là thư viện mới của Google để đào tạo Rừng quyết định.
YDF mở rộng sức mạnh của TF-DF, cung cấp các tính năng mới, API đơn giản hóa, thời gian đào tạo nhanh hơn, tài liệu cập nhật và nâng cao khả năng tương thích với các thư viện ML phổ biến.
Rừng quyết định TensorFlow ( TF-DF ) là thư viện để đào tạo, chạy và giải thích các mô hình rừng quyết định (ví dụ: Rừng ngẫu nhiên, Cây tăng cường độ dốc) trong TensorFlow. TF-DF hỗ trợ phân loại, hồi quy, xếp hạng và nâng cao.
Từ khóa: Rừng quyết định, TensorFlow, Rừng ngẫu nhiên, Cây tăng cường độ dốc, CART, diễn giải mô hình.Tài liệu & Tài nguyên
Các tài nguyên sau đây có sẵn:
- Hướng dẫn và hướng dẫn
- Tham chiếu API
- Tài liệu YDF (cũng áp dụng cho TF-DF)
- Lớp học trực tuyến về Nhóm quyết định của Nhà phát triển Google