使用 JavaScript 进行 TensorFlow 开发
在开始学习下面的学习资料之前,您应该:
-
对使用 HTML、CSS 和 JavaScript 进行浏览器编程有所了解
-
熟悉如何使用命令行运行 Node.js 脚本
本课程适用于想要实现以下目标的人员:
-
用 JavaScript 构建机器学习模型
-
在能运行 JavaScript 的任何环境中运行现有模型
-
将机器学习模型部署到网络浏览器中
借助 TensorFlow.js,您可以使用 JavaScript 开发或执行机器学习模型,并直接在浏览器客户端中使用机器学习技术,或者通过 Node.js、React Native、Electron,甚至是 Raspberry Pi 上的 Node.js,在服务器端、移动原生设备、桌面原生设备以及 IoT 设备中使用机器学习技术。如需详细了解 TensorFlow.js 及其作用,请观看 Google I/O 大会上的这段演讲视频。
第 1 步:了解浏览器中的机器学习
如需大致了解使用 JavaScript 进行机器学习的基础知识,请自学 Edx 上的课程,也可以观看以下视频,从基本原理开始,学习如何使用现有的预构建模型,甚至构建您自己的分类神经网络。您还可以试试使用 JavaScript 构建智能摄像头 Codelab,通过交互式演示教程了解这些概念。
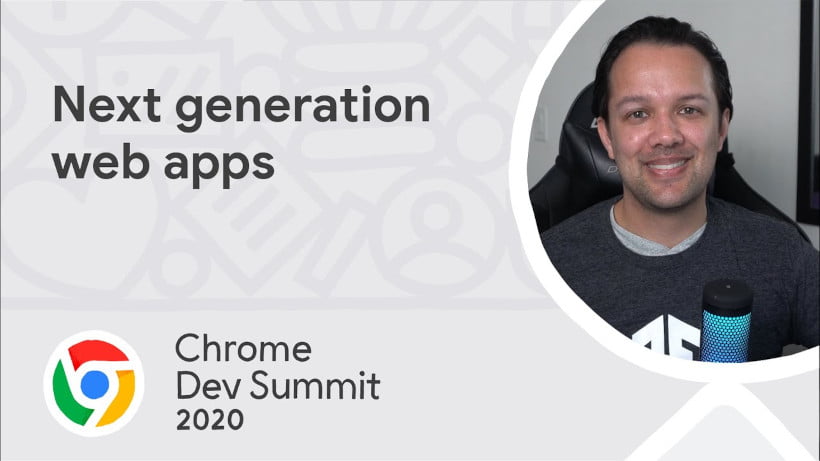
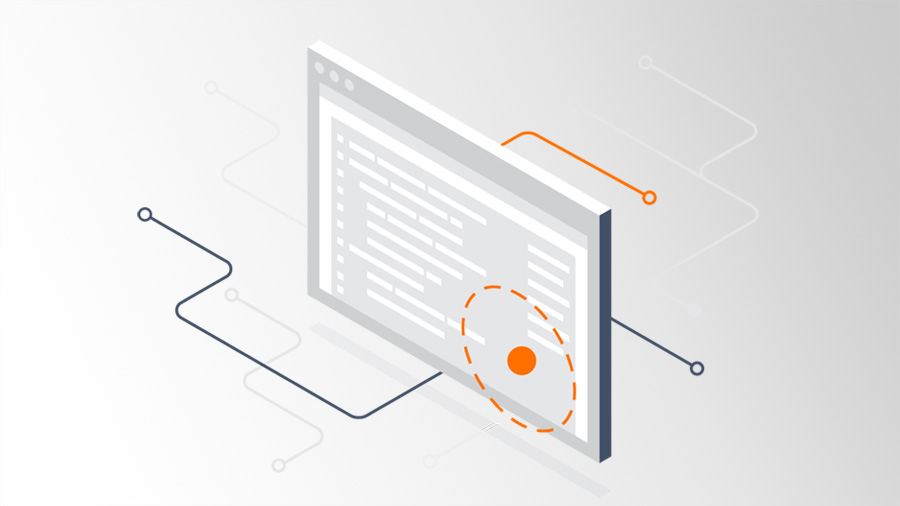
借助 TensorFlow.js,从 Web 机器学习新手成为高手。了解如何创建新一代可在客户端运行且能在几乎所有设备上使用的 Web 应用。
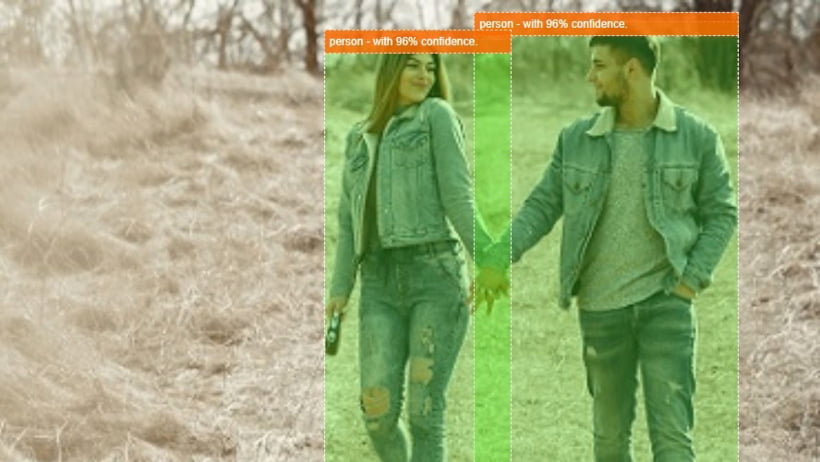
了解如何加载和使用一种 TensorFlow.js 预训练模型 (COCO-SSD),并用它来识别训练模型时所用到的常见对象。
第 2 步:更加深入地了解深度学习
为帮助您更加深入地了解神经网络的工作原理,以及更加全面地了解如何运用神经网络解决各种问题,我们向您推荐两本书。
如果您基本上刚开始接触张量和机器学习,但非常了解 JavaScript,《学习 TensorFlow.js》一书是入门的理想之选。本书将全面介绍各种知识,从了解如何以张量形式操纵数据等基础知识开始,一直到快速应用到实际应用中。阅读本书后,您将了解如何加载现有模型、将数据传递给这些模型以及解读产生的数据。
《使用 JavaScript 进行深度学习》一书也是入门的理想之选。其中附带了 GitHub 上的大量示例,可供您练习使用 JavaScript 进行机器学习。
这本书介绍了如何使用各种神经网络架构(例如卷积神经网络、循环神经网络)和高级训练范例(例如强化学习),另外也清楚解释了神经网络在训练过程中实际发生的情况。
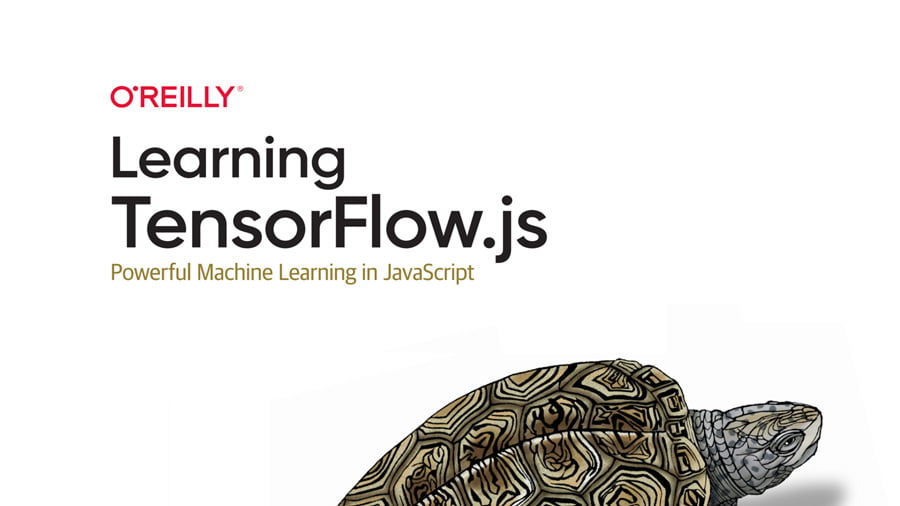
通过端到端的实际操作方法学习 TensorFlow.js 基础知识,适合广泛的技术受众。读完本书后,您将了解如何使用 TensorFlow.js 构建和部署可用于生产环境的深度学习系统。
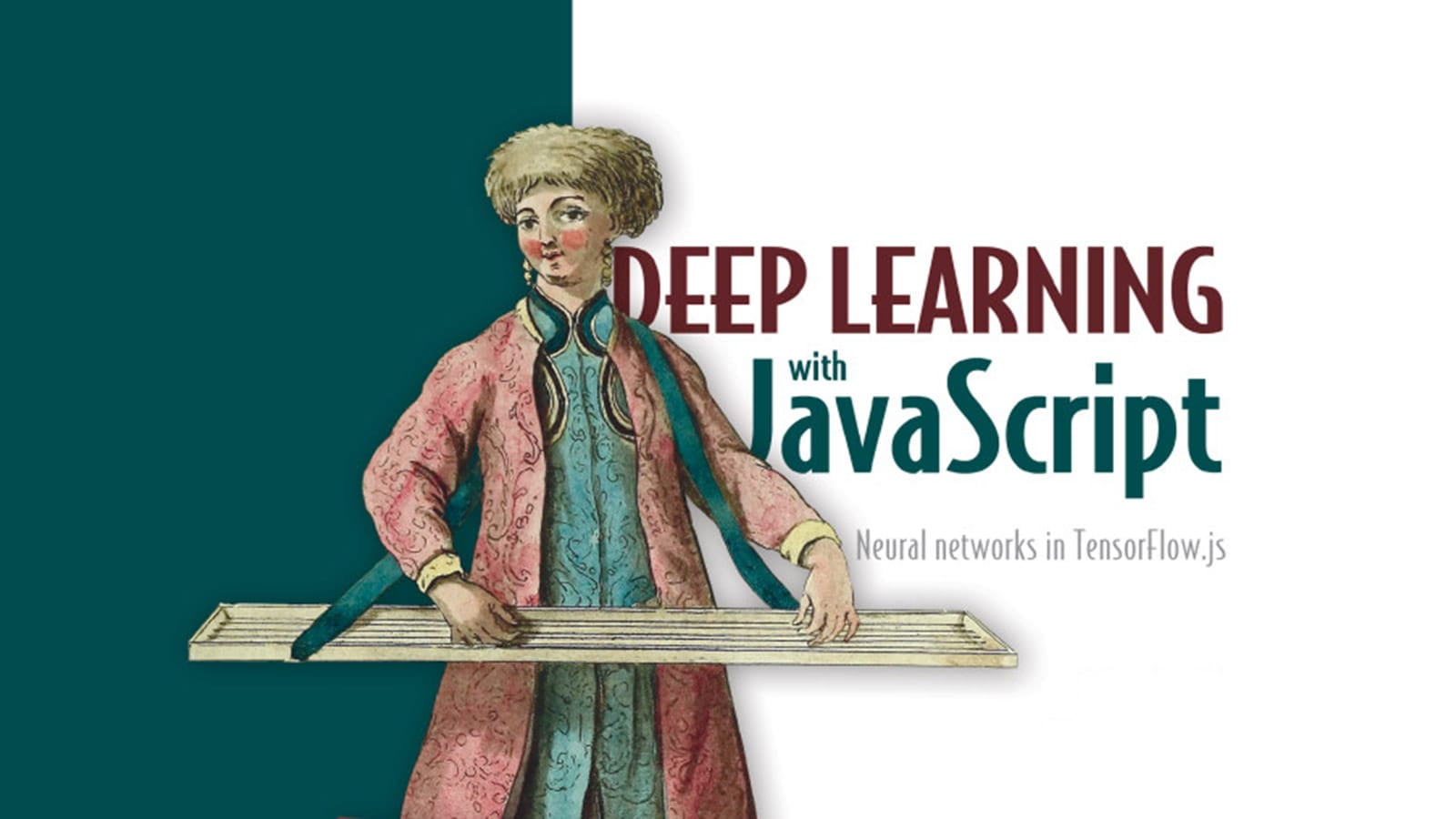
本书由 TensorFlow 库的主要作者编著,提供了关于在浏览器中或 Node 上使用 JavaScript 构建深度学习应用的精彩用例和深入说明。
第 3 步:使用 TensorFlow.js 进行示例练习
熟能生巧,亲身体验是掌握概念的最佳方式。请查看 TensorFlow.js Codelab,了解针对常见使用场景的分步指南,加深对知识的理解:
对神经网络有所了解之后,您可以更加轻松地探索由 TensorFlow 团队创建的开源示例。所有示例均可在 GitHub 上找到,因此您可以深入了解代码及其工作原理。
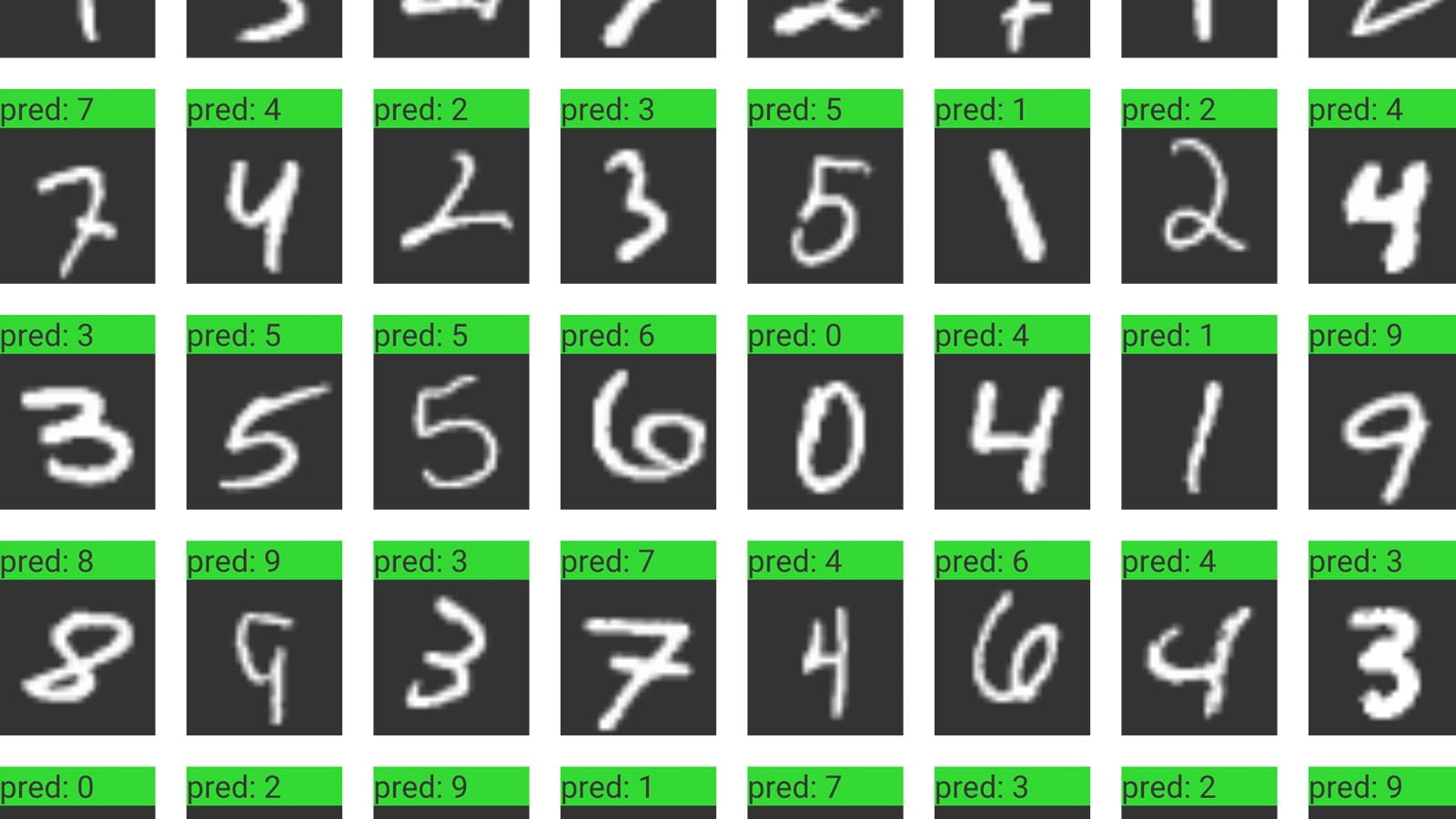
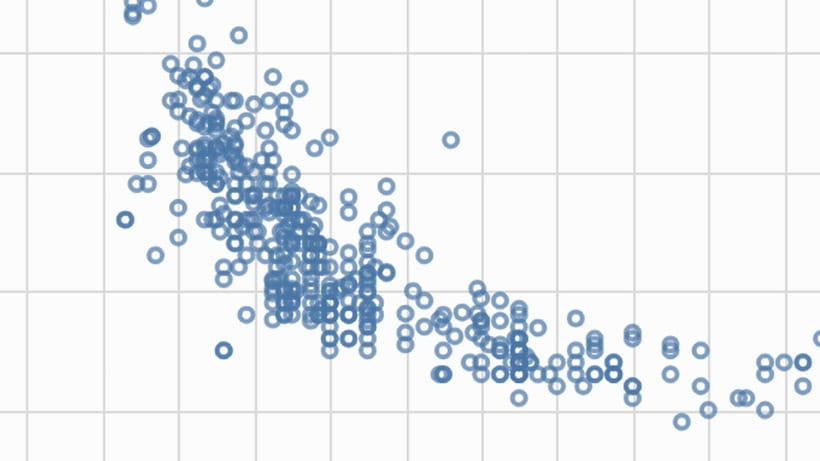
TensorFlow 教程是以 Jupyter 笔记本的形式编写而成,可直接在 Google Colab(一种无需设置的托管式笔记本环境)中运行。点击“在 Google Colab 中运行”按钮。
第 4 步:创建新项目!
在检验了知识掌握情况并使用一些 TensorFlow.js 示例练习之后,您应该就可以开始开发自己的项目了。参考我们的预训练模型,马上就可以开始构建应用。或者,您也可以使用收集的数据或使用公共数据集训练自己的模型。Kaggle 和 Google 数据集搜索是查找用于训练模型的开放数据集的理想之地。
如果您在寻找灵感,不妨观看我们的 Made With TensorFlow.js 之 Show and Tell 剧集,了解世界各地的开发人员如何在其应用中使用 TensorFlow.js。
您也可以在社交媒体上搜索 #MadeWithTFJS # 标签,查看来自社区的最新贡献内容。