تعلم الآلة مرن ومتحكم فيه وقابل للتفسير مع نماذج قائمة على الشبكة
import numpy as np import tensorflow as tf import tensorflow_lattice as tfl model = tf.keras.models.Sequential() model.add( tfl.layers.ParallelCombination([ # Monotonic piece-wise linear calibration with bounded output tfl.layers.PWLCalibration( monotonicity='increasing', input_keypoints=np.linspace(1., 5., num=20), output_min=0.0, output_max=1.0), # Diminishing returns tfl.layers.PWLCalibration( monotonicity='increasing', convexity='concave', input_keypoints=np.linspace(0., 200., num=20), output_min=0.0, output_max=2.0), # Partially monotonic categorical calibration: calib(0) <= calib(1) tfl.layers.CategoricalCalibration( num_buckets=4, output_min=0.0, output_max=1.0, monotonicities=[(0, 1)]), ])) model.add( tfl.layers.Lattice( lattice_sizes=[2, 3, 2], monotonicities=['increasing', 'increasing', 'increasing'], # Trust: model is more responsive to input 0 if input 1 increases edgeworth_trusts=(0, 1, 'positive'))) model.compile(...)
TensorFlow Lattice هي مكتبة تنفذ نماذج شبكية مقيدة وقابلة للتفسير. تمكنك المكتبة من إدخال معرفة المجال في عملية التعلم من خلال قيود الشكل المنطقية أو القائمة على السياسة. يتم ذلك باستخدام مجموعة من طبقات Keras التي يمكنها تلبية قيود مثل الرتابة والتحدب وكيفية تفاعل الميزات. توفر المكتبة أيضًا نماذج سهلة الإعداد.
باستخدام TF Lattice، يمكنك استخدام معرفة المجال لاستقراء أفضل لأجزاء مساحة الإدخال التي لا تغطيها مجموعة بيانات التدريب. يساعد هذا في تجنب سلوك النموذج غير المتوقع عندما يختلف توزيع الخدمة عن توزيع التدريب.
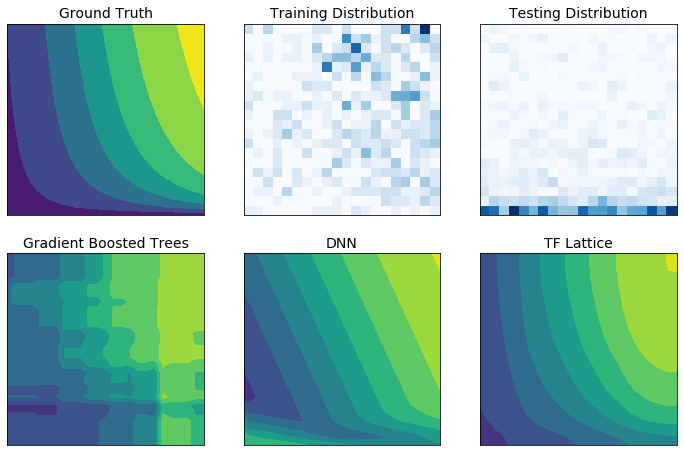