TensorFlow 的文本处理工具
import tensorflow as tf import tensorflow_text as tf_text def preprocess(vocab_table, example_text): # Normalize text tf_text.normalize_utf8(example_text) # Tokenize into words word_tokenizer = tf_text.WhitespaceTokenizer() tokens = word_tokenizer.tokenize(example_text) # Tokenize into subwords subword_tokenizer = tf_text.WordpieceTokenizer( lookup_table, token_out_type=tf.int64) subtokens = subword_tokenizer.tokenize(tokens).merge_dims(1, -1) # Apply padding padded_inputs = tf_text.pad_model_inputs(subtokens, max_seq_length=16) return padded_inputs
TensorFlow 为您提供一系列丰富的操作和库,帮助您处理文本形式的输入,例如原始文本字符串或文档。这些库可以执行基于文本的模型经常需要进行的预处理,并且包含对序列建模非常有用的其他功能。
您可以从 TensorFlow 图内部提取强大的语法和语义文本特征,并将这些特征作为神经网络的输入。
将预处理与 TensorFlow 图集成在一起可提供以下优势:
- 有助于使用大型工具包处理文本
- 允许与一套丰富的 TensorFlow 工具集成,以便为项目提供支持,包括问题定义、训练、评估和发布等
- 降低应用模型时的复杂性,并防止出现训练-应用偏差
除上述各项外,您无需担心训练中的分词化与推断时的分词化不同,也无需担心如何管理脚本的预处理。
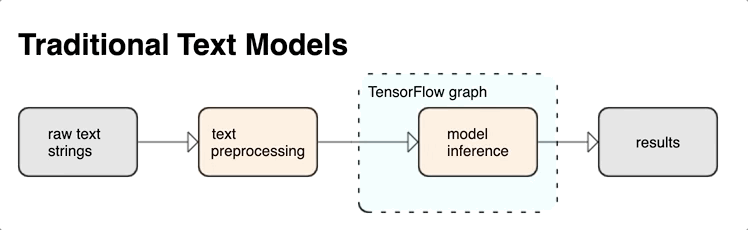